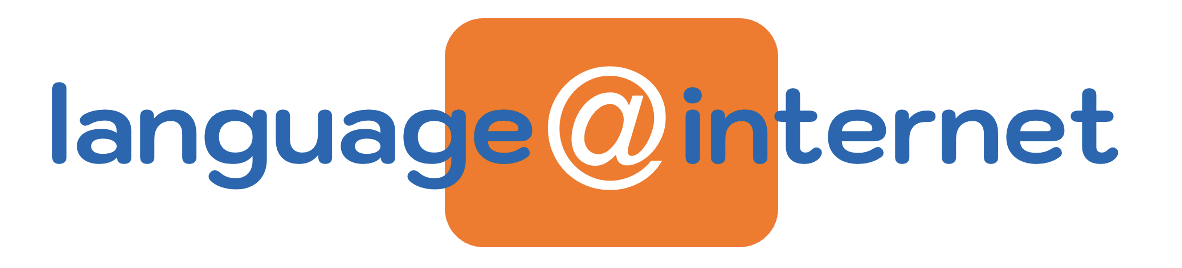
Language@Internet is an open-access, peer-reviewed, scholarly electronic journal that publishes original research on language and language use mediated by the Internet, the World Wide Web, and mobile technologies. ISSN: 1860-2029
Current Issue
Vol. 21 (2023)
Published: 2023-12-30
Articles
==========================================================================================
Language@Internet is listed in Cabells Journalytics, a searchable, curated database of reputable international scholarly publications.